Organizations are always looking for effective methods to enhance efficiency and discover new possibilities, with generative AI proving to be a powerful tool for achieving these objectives. This technology creates new content, from realistic visuals to personalized text, by analyzing patterns in data. In this article, we explore how Generative AI works, focusing on the neural networks and deep learning techniques that power its capabilities. Learn about its diverse applications, including streamlining content creation, enhancing customer engagement, and driving product innovation.
Understanding Generative AI: The Basics
Before we get into the details, we should determine what the term generative AI actually means. In this paragraph you will find the definition and key components of this technology.
What Is Generative AI?
At its core, generative AI refers to algorithms that can produce new content rather than simply analyze or process existing information. These are models designed to understand and generate multimodal content, mimicking human creativity through machine learning and neural networks. The rise of genAI apps, like local ChatGPT or AI-powered knowledge base, has shown its potential in delivering unique and tailored outputs in various formats.
Key Components
GenAI has multiple key components that allow this system to be as effective as it is. Here are some of them:
- Machine Learning Algorithms: These algorithms enable the model to learn from large datasets, improving its capacity to generate new content.
- Neural Networks: Algorithms inspired by the structure and functioning of the human brain that learn to recognize patterns in data. Thanks to the use of layers of neurons and advanced optimization techniques, these networks are capable of generating realistic and creative results that often resemble works created by humans.
- Training Data: Vast amounts of data that the algorithm uses to learn patterns and styles, helping it to create coherent and contextually relevant outputs.
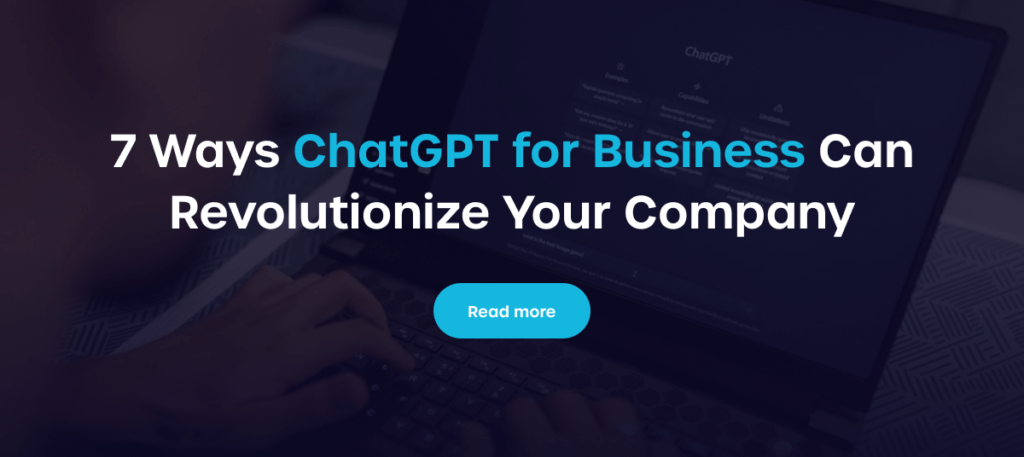
How Does Generative AI Work?
Generative AI operates on the principles of analysis and creation, leveraging complex models to emulate human-like cognition. The process involves multiple stages that include data ingestion, machine learning, and ultimately, content generation. This mechanism underpins the capabilities found in many apps, including those offering AI-driven solutions.
The Science Behind Generative AI
At the heart of generative AI lies deep learning, where neural networks learn to represent vast amounts of data through various layers of abstraction. These networks make predictions or generate data that adheres to learned patterns, enabling them to create remarkably sophisticated outputs. Essentially, the technology gives machines the ability to ‘think’ and produce creatively, a feat that was once thought to be exclusive to humans.
Examples of Processes
The technology we refer to can perform a wide range of tasks. Here are some of the most important and widely used examples:
- Text Generation: Creating original dialogue or prose based on a prompt.
- Image Synthesis: Transforming concepts into visual art through algorithms like GANs (Generative Adversarial Networks).
- Style Transfer: Applying the style of one image to the content of another, producing a blended artwork.
Do you want to create your own generative AI application?
Where Can Generative AI Be Used?
Generative AI App Development encompasses a variety of products, spanning from creative fields to practical business solutions. Its ability to generate new ideas has made it particularly appealing in the arts, while its efficiency offers impressive opportunities in industrial applications and technology. As this field continues to advance, its reach is likely to expand even further.
Generative AI in Creative Industries
In the creative sector, generative AI tools are being harnessed to innovate in music, art, and writing. For example, artists are using genAI to inspire new styles and techniques, while authors utilize AI-generated content for inspiration and plot development. This integration not only enhances artistic expression but also allows creators to explore concepts that would be challenging to visualize without artificial intelligence support.
Examples of such applications include VocaliD and Synthesia, both leveraging AI-driven solutions to generate content. The first app specializes in creating natural voice personas, while the second platform transforms text into videos within minutes.
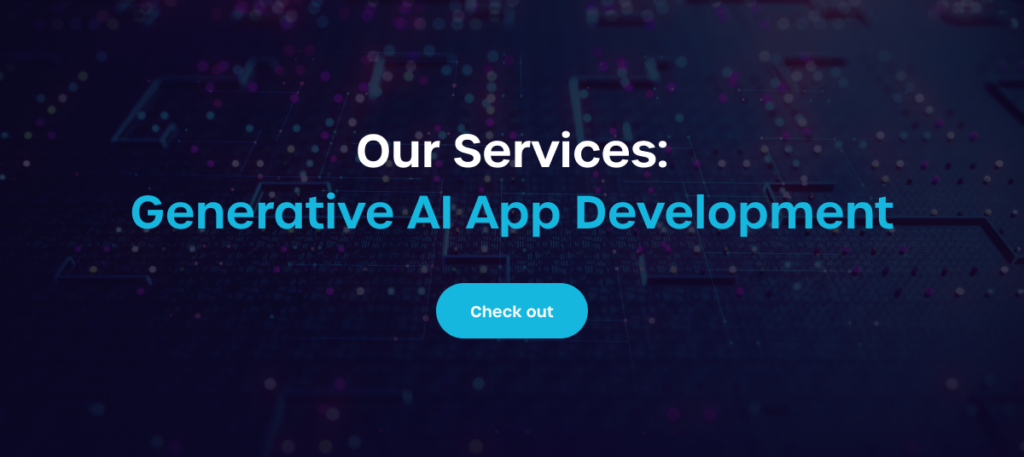
GenAI in Business and Technology
Another sector that can really benefit from genAI is business and technology. Artificial Intelligence applications, such as internal ChatGPT, can generate content that can help employees and customers:
- Data Analysis and Recommendations: AI-powered knowledge base can analyze client data and generate actionable business insights, streamlining operations and improving decision-making.
- Customer Service Automation: By integrating local chatGPT functionalities, companies can offer real-time support with personalized responses.
Do you want to create your own AI-powered knowledge base? Check out our product:
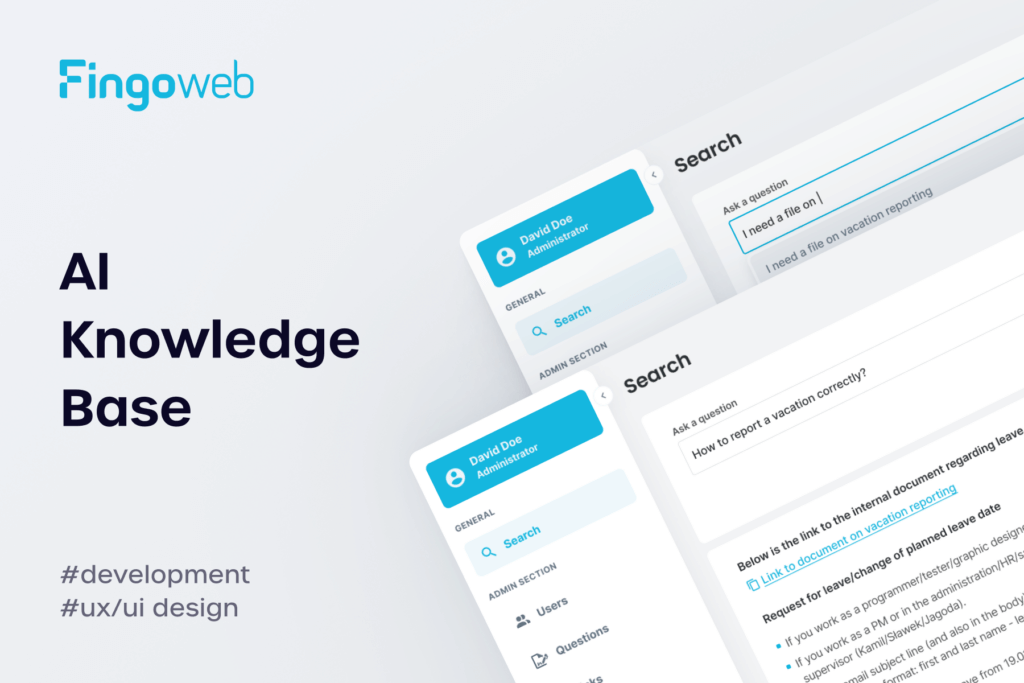
Other Examples
Generative AI is also advancing education through personalized learning, with AI-driven solutions that adapt content to meet the unique needs of each student. Additionally, gaming industries use genAI to create dynamic and immersive environments, enhancing player experiences. Furthermore, sectors like healthcare are exploring the technology for predictive modeling and diagnostics.
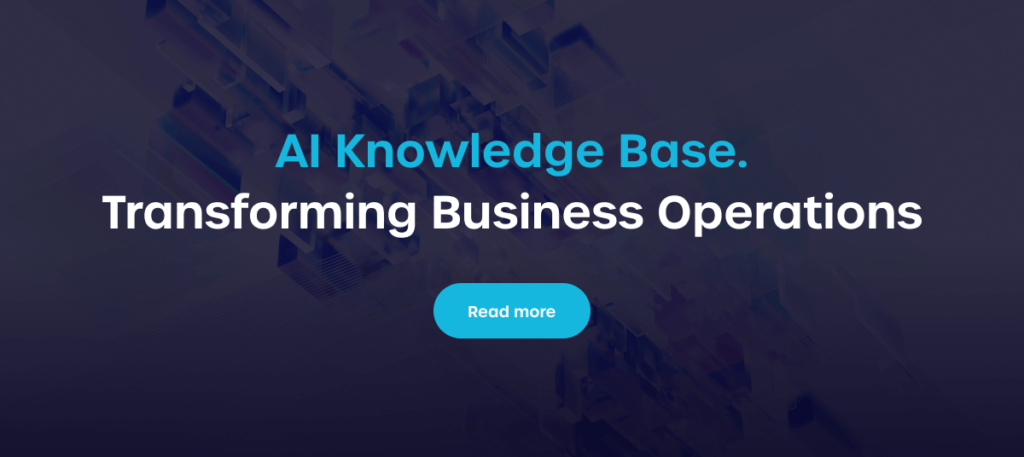
Challenges and Future
As with any emerging technology, generative AI is not without its challenges. Ethical considerations surrounding the authenticity of generated content and the potential for misuse highlight the need for robust governance frameworks. Addressing these challenges is essential for maximizing the benefits while mitigating risks.
Challenges in GenAI
Like every other modern innovation, generative AI brings some challenges to the table. Here is what raises the most doubts:
- Ethical Dilemmas: Concerns over plagiarism and ownership arise when AI-generated content closely resembles human-created work.
- Bias in Training Models: The models may inadvertently march existing biases in their training data, leading to skewed or harmful outputs.
- Technological Limitations: Despite impressive capabilities, generative AI still struggles with understanding context and nuance, which can lead to frustrating user experiences.
The Future of Generative AI
The future of genAI holds enormous promise across various sectors. With advancements in technology, we can expect increased efficiency and more nuanced outputs that can potentially revolutionize industries. As the dialogue around governance and ethical implications evolves, the integration of genAI into everyday applications, such as internal chatGPT, will likely become commonplace, fundamentally altering how we interact with machines.
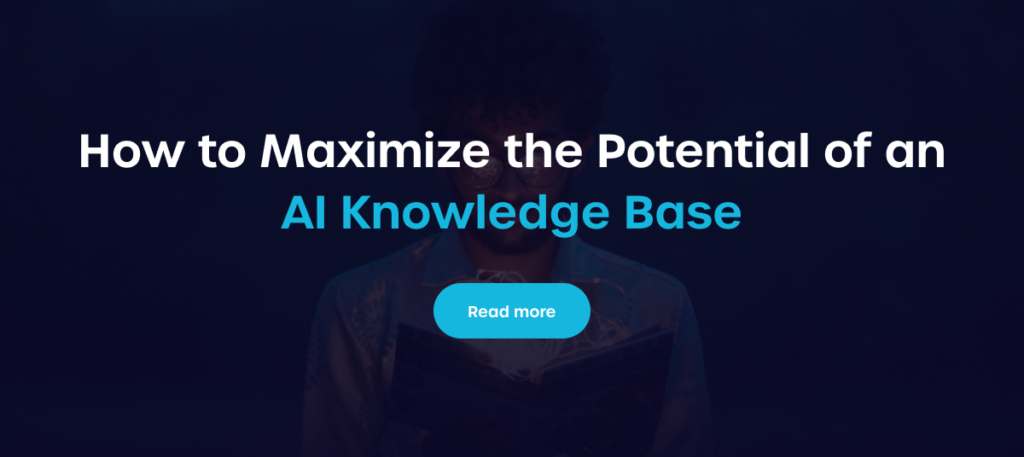
FAQ
What is the Definition of Generative AI?
Generative AI refers to artificial intelligence systems designed to create new and original content, such as images, text, music, or code, by learning patterns from existing data. It uses advanced techniques like deep learning to generate outputs that mimic human-like creativity. Unlike traditional AI, which focuses on analyzing and predicting, genAI actively produces unique outputs based on the data it has been trained on. Examples of apps using this technology include an internal chatGPT or an AI-powered knowledge base.
Is Generative AI Safe?
Generative AI is generally safe when used responsibly, but it comes with risks that depend on how it’s implemented and monitored. Potential concerns include the creation of misleading content, ethical misuse, or biases inherited from training data. Ensuring safety involves strict oversight, robust guidelines, and transparency in its development and application to minimize harm and maximize benefits.
How Can I Implement GenAI in My Company?
Implementing Generative AI in your company starts with identifying tasks that can benefit from automation or enhanced creativity, such as content generation, customer support, or product design. Choose reliable AI tools or platforms that align with your business goals and integrate them into existing workflows, ensuring proper training for your team. To maximize success, monitor performance regularly, address ethical considerations, and maintain compliance with data security and privacy standards.